The Crucial Role of Artificial Intelligence in Diagnosing Diseases
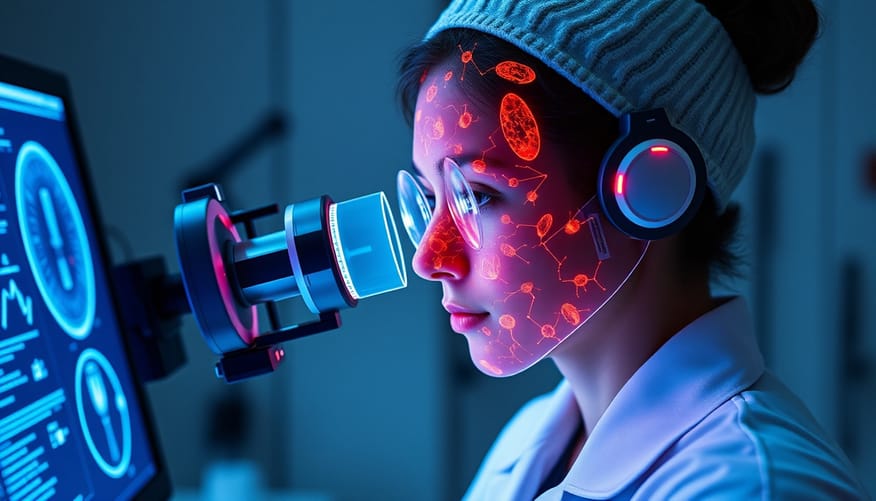
The Evolution of Diagnostic Technologies
The medical field has witnessed tremendous advancements over the decades, particularly in diagnostic technologies. Historically, diagnosis relied heavily on the expertise of physicians and observable symptoms. With the advent of tools like X-rays, CT scans, and MRIs, the ability to diagnose accurately improved significantly. However, with the rise of Artificial Intelligence (AI), we stand on the brink of another revolutionary transformation in diagnostics.
Understanding AI in Medical Diagnostics
AI in medical diagnostics involves the use of complex algorithms and machine learning to interpret complex medical data. These algorithms can analyze images, physiological signals, and patient records faster and in some cases, more accurately than traditional methods. AI applications range from image recognition, predicting disease outcomes, to automating routine tasks, broadening the horizons of medical professionals by offering greater insights.
AI Technologies Powering Diagnostics
The primary driving forces are Machine Learning (ML), Natural Language Processing (NLP), and deep learning. ML algorithms process vast amounts of data and identify patterns beyond human capability. NLP helps in understanding and interpreting verbal interaction, while deep learning simulates the human brain's neural networks, enhancing pattern recognition in images and videos, crucial for diagnostics like radiology and pathology.
AI's Impact on Diagnostic Accuracy
One of the most significant contributions of AI in diagnostics is its ability to enhance accuracy and reduce human error. Machines can consistently analyze patterns in data, making them invaluable in settings such as radiology where vast numbers of images need to be examined meticulously. Studies have shown that AI systems can match, or even exceed, human doctors in diagnosing certain conditions like breast cancer from mammograms.
Case Studies and Success Stories
Several case studies demonstrate the successful application of AI in diagnostics. For instance, IBM's Watson has been used in oncology to comb through massive amounts of medical literature and patient data to suggest treatment options, enhancing personalized medicine. Another example is Google's DeepMind, which has developed AI capable of diagnosing eye diseases with an accuracy comparable to professional ophthalmologists.
Challenges and Limitations
Despite the promising potential, AI in diagnostics faces several challenges. Data privacy concerns are paramount, as AI systems need extensive patient data to be effective. Furthermore, the interpretation of AI recommendations in clinical settings requires careful oversight. Another major concern is the integration of AI technologies into existing healthcare systems, which often require substantial infrastructural updates.
The Future of AI in Diagnosing Diseases
Looking ahead, AI is expected to become even more integrated with diagnosing diseases, offering more personalized and predictive healthcare. The future may see AI-driven wearable devices providing real-time health monitoring and diagnostics, potentially transforming how we approach health and disease management.
Conclusion: Embracing an AI-Enhanced Healthcare
AI is undoubtedly transforming the landscape of medical diagnostics. As the technology matures, healthcare providers stand to benefit from greater precision, efficiency, and personalized insights, ultimately leading to improved patient outcomes. Embracing these technological advancements while addressing ethical and operational challenges will be vital for the future of healthcare.