Advancements in Natural Language Processing: Bridging the Communication Gap
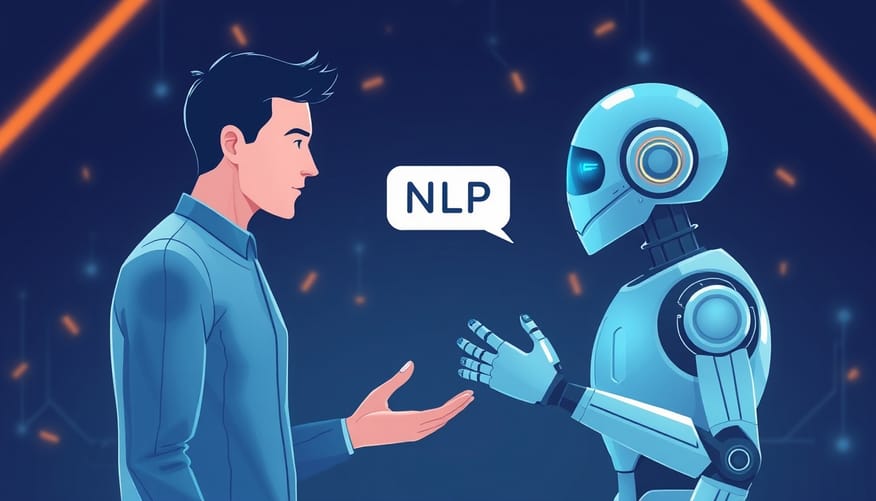
Introduction to Natural Language Processing
Natural Language Processing (NLP) is a field of artificial intelligence that focuses on the interaction between computers and humans using the natural language. The ultimate objective of NLP is to enable computers to understand, interpret, and generate human languages in a valuable way. Recent advancements in NLP have significantly enhanced the way machines process language, bridging the communication gap between humans and machines.
The Evolution of NLP Methods
NLP has come a long way from its inception. Early methods relied heavily on rule-based systems, which involved manually defining linguistic rules. These were succeeded by statistical models that leveraged linguistic corpora. However, the real breakthrough came with machine learning, particularly deep learning models, which allowed machines to understand language in context rather than isolated words. Techniques like BERT and GPT-3 have revolutionized the field, enabling tasks like translation, summarization, and sentiment analysis with unprecedented accuracy.
Key Technologies Driving NLP Advancements
The advancements in NLP have been driven by several key technologies. Machine learning and deep learning play a pivotal role, allowing machines to learn from vast amounts of data. Transfer learning and pre-trained models like BERT have set new benchmarks in understanding context. Additionally, reinforcement learning is enhancing the ability of NLP systems to perform tasks autonomously by learning from their actions.
Real-World Applications of Advanced NLP
The real-world applications of NLP are vast and growing rapidly. In customer service, chatbots and virtual assistants like Siri and Alexa have made communication with technology seamless. NLP is also transforming industries like healthcare, where it helps in processing medical records and aiding diagnostics. In finance, it's used for predicting market trends and managing risks. As NLP technology evolves, its applications in everyday life continue to expand.
Challenges in NLP and Future Directions
Despite significant advancements, NLP still faces several challenges. Understanding context, especially sarcasm and idioms, in different languages remains complex. There's also the issue of bias in AI, as models trained on biased data can perpetuate prejudice. Looking ahead, researchers are focusing on making NLP models more generalized and bias-free. Enhancements in model efficiency and inclusivity are key to unlocking the full potential of NLP.
Conclusion
Advancements in natural language processing continue to close the communication gap between humans and machines, making our interactions more intuitive and natural. As technologies improve, the potential for NLP applications is vast, promising enhancements in both personal and professional spaces. Continued research and innovation in this field will further bridge communication barriers, paving the way for a future where interacting with technology feels as natural as conversing with another human.